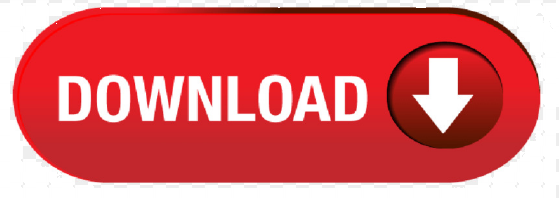

In this study, we only use grayscale malware images from a modified ResNeXt to analyze the malware samples. Furthermore, we evaluated the effectiveness of the modified ResNeXt model in the classification process by testing a total of fifteen different combinations of the Android malware image sections on the Drebin dataset. Using DL techniques not only avoids the domain expert costs but also eliminates the frequent need for the feature engineering methods.

In addition, we applied fine-tuned deep learning (DL) based on the convolutional neural network (CNN) on the visualized malware samples to automatically obtain the discriminatory features that separate normal from malicious data. The nonintuitive malware's features are converted into fingerprint images in order to extract the rich information from the input data. In addition, we present a comprehensive evaluation of the Android malware classification and detection using our modified ResNeXt. In this study, we proposed a modified ResNeXt model by embedding a new regularization technique to improve the classification task. The categorization of malware families may be used to improve the efficiency of the malware detection process as well as to systematically identify malicious trends. Identifying and categorizing dangerous applications into categories that are similar to one another are especially important in the development of a safe Android app ecosystem. It is critical to successfully identify, mitigate, and fight against Android malware assaults, since Android malware has long been a significant threat to the security of Android applications.
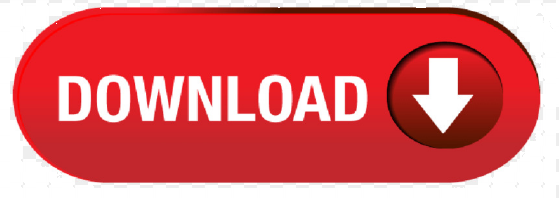